Improving Indicators for Tumor Diagnosis
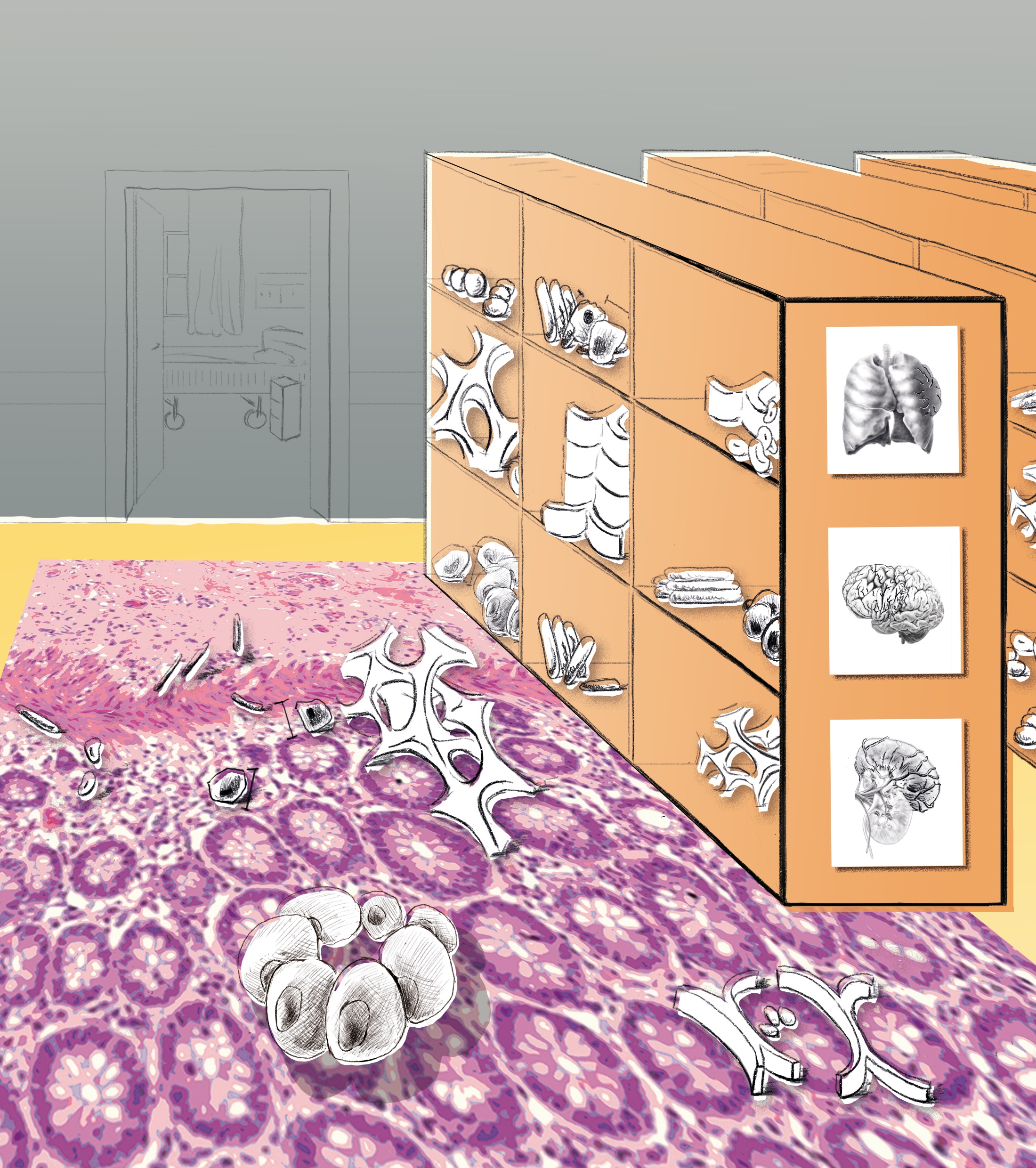
Biomarkers are often indispensable for accurate diagnoses. The Fraunhofer Institute for Digital Medicine MEVIS is working on adaptive algorithms that make the search for new biomarkers much easier. This can provide physicians with valuable support in choosing the best possible therapy.
Biomarkers make up an important building block of diagnostics. One common example is the cholesterol level in blood, which can suggest an increased risk of cardiovascular disease. Biomarkers also play a significant role in pathology, the microscopic examination for example of suspected tumor tissue samples. If particular cell types with certain combinations of properties are present, this can be considered a meaningful indicator that ideally reveals which subtype of tumor is present. This lets physicians select a targeted treatment that is effective for each individual patient.
In clinical practice, however, this accurate procedure does not always work. A study from 2018, for example, revealed that 44 percent of cancer patients in the United States should be considered for immunotherapy, a special type of tumor treatment. In reality, however, this treatment was only effective in 12 percent of patients, meaning that many were treated in vain. “To provide more targeted therapy in the future, we need to be able to subdivide tumor types much more precisely than at the present,” says MEVIS researcher Johannes Lotz. “We need to discover the biomarkers that deliver this differentiation.”
Detecting such biomarkers demands extensive clinical studies. The adaptive AI systems of the future will assist in this search. “The computer analyzes digitalized tissue sections and looks through them for patterns,” says Lotz’s colleague Henning Höfener when describing the strategy. “This enables it to find new biomarkers.” The software must be trained with as many sets of high-quality data as possible, otherwise the search for patterns will be unsuccessful. This creates a problem: The more precise the differentiation between different tumor subtypes, the fewer patients exhibit a certain subtype and the fewer data sets are available for training and analysis.
The Best of Two Worlds
Another difficulty: “The appearance of digital tissue sections can vary significantly from laboratory to laboratory,” explains Lotz. “This makes it difficult for the computer to detect existing patterns in the images reliably.” These problems can hardly be overcome using previous AI methods, in which the algorithms sift through vast quantities of pixels. That’s why the MEVIS team is attempting a new strategy and is taking a look at some of the tried-and-true ways in which humans work. “Experienced pathologists have seen thousands and thousands of tissue images and derive the essential laws from them,” explains Höfener. “Unlike AI, a handful of images is typically sufficient for an accurate diagnosis.”
The MEVIS team wants to bring the best of both worlds together. Their plan is to train the artificial intelligence as if it were a pathologist, using many tissue images unrelated to a specific inquiry. Thanks to this “basic training,” the AI acquires knowledge about general characteristics and relations, so-called tissue descriptors. With their help, the machine can describe and classify the images. “If the algorithm then encounters a specific problem, it can use tissue descriptors to find correlations. Even with relatively few data, this could predict, for example, the success of a certain therapy,” says Höfener.
The project is still in its beginning phase, but Fraunhofer MEVIS is well prepared to master it successfully. “We have a lot of experience training adaptive algorithms and programming computer-aided diagnostic tools,” emphasizes Henning Höfener. “In cooperation with our clinical project partners, we can select the data we need for initial algorithm training.”
AI Accelerates Automatic Detection
These biomarker algorithms will benefit the research departments of pharmaceutical companies, but also university workgroups. The approach promises additional applications, however. For example, tissue descriptors could be used for segmentation, the automatic recognition and measurement of an image’s tissue structures. “The descriptors also reduce the amount of training data required in this case,” says Höfener. “This could significantly accelerate adaptation of the segmentation algorithm.”
Content-based image retrieval could also benefit from this method. “Many are familiar with a similar feature on Google, where an image can be uploaded and similar ones are displayed,” explains Johannes Lotz. “For medical images, however, this is not yet as reliable.” The MEVIS experts hope that the descriptor concept could lead to substantial advancements. They envision a system that allows physicians who encounter unusual findings to search databases for similar images to check their diagnoses and discover which therapies have or have not worked in the past.