Guidepost for the Best Choice of Treatment
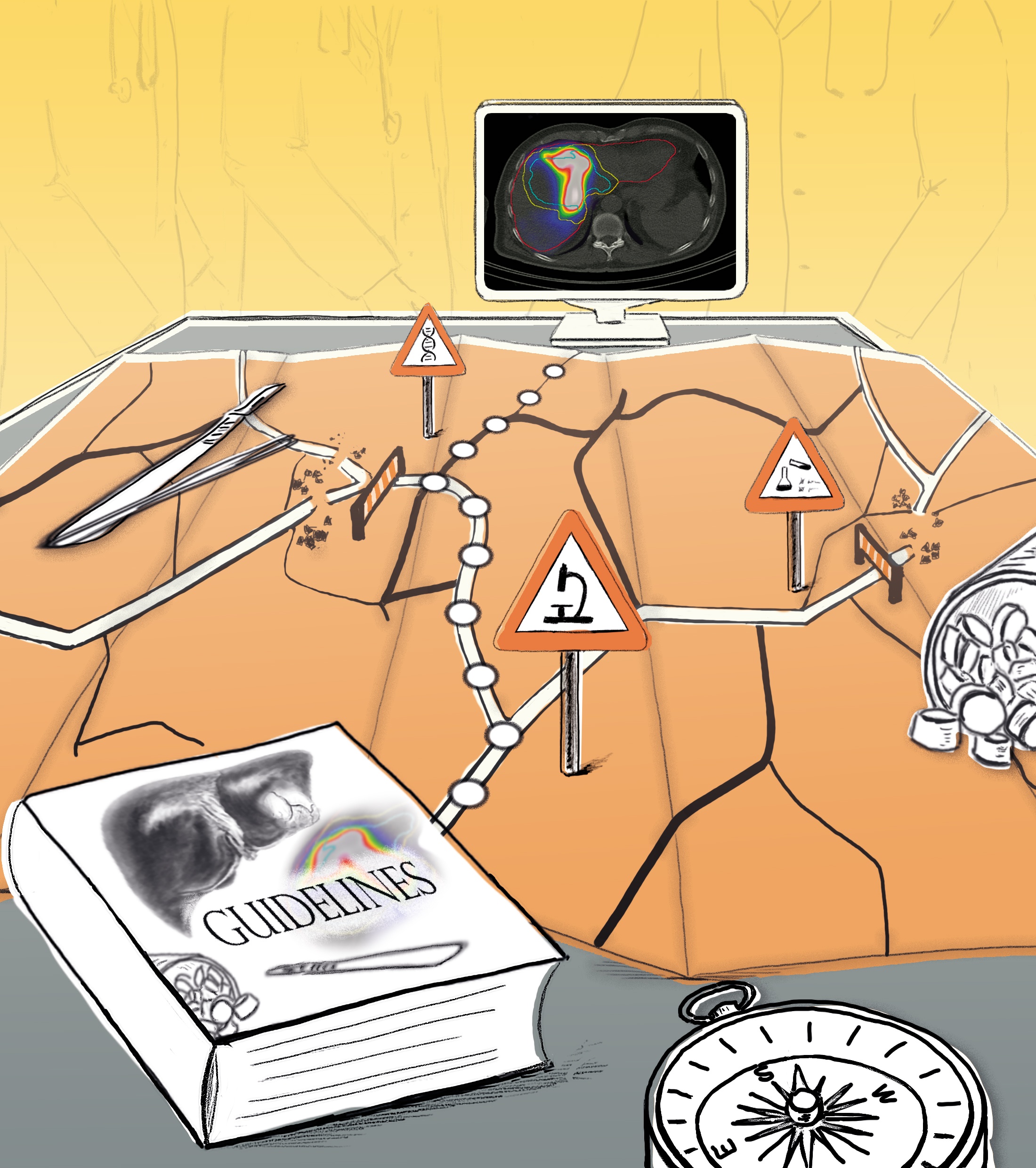
A diagnosis has been made. Now it is up to clinicians, together with their patients, to find a suitable treatment. However, as more and more treatment choices are becoming available, the most suitable one isn’t always the most obvious choice. The Fraunhofer Institute for Digital Medicine MEVIS is developing AI-based support systems to facilitate decision-making and pave the path towards finding the optimal treatment.
Operate or wait? Chemotherapy or not? Stay on the current medication or change to another? These decisions constitute the everyday routine in clinics and medical practices. These decisions are, however, not always easy to make: “A treatment decision usually depends on a variety of factors,” says Fraunhofer MEVIS researcher Andrea Schenk. “What image data is available? Does the patient have a pre-existing condition? What are our treatment options? What do the corresponding guidelines recommend?” Making these decisions is challenging because medical progress is constantly increasing both the amount of data available and the number of treatment choices.
This is where CDS (clinical decision support) systems can help. Ideally, these decision support systems characterize the patient as an individual digital model incorporating all available data and current guidelines. The software can simulate and compare various treatment approaches. This gives physicians important clues as to which treatment is most effective or gentle for the patient. “Until now, only basic CDS systems have been available that are limited to specific subdomains,” explains Schenk’s colleague Markus Wenzel. “Now, we are working on a broader solution that will incorporate and unify data from an wide range of sources.”
Filling gaps in the guidelines
The core notion is that novel systems should act individually for each patient instead of according to a relatively rigid scheme. A guideline might, for instance, recommend that a patient with breast cancer undergo a mastectomy. In contrast, a CDS system fed with extensive image and laboratory data of the patient and the results of recent clinical studies might reach a different conclusion and recommend breast-preserving radiotherapy for better results.
“Guidelines can never cover all cases and, thus, inevitably have gaps,” explains Wenzel. “We aim to fill these gaps with the help of intelligent modeling.” An important element of this is segmentation, for which software can, for example, automatically detect the size and location of a tumor on an MR image. Segmentation forms the foundation for creating an individual patient model.
“We can simulate the success or failure of a planned liver intervention or tumor sclerotherapy based on using this model as a basis,” explains Andrea Schenk. “We have already achieved a lot of groundwork in these areas, which we can integrate into future systems.” After all, Fraunhofer MEVIS is not in the position to build a complete CDS system alone. Instead, the institute will deliver core components and work alongside a tight network of research partners, companies, and professional associations.
Transparent Algorithms
Future CDS systems will include additional elements, such as adaptive algorithms, to detect cases in medical databases that progressed similarly. This comparison could provide the attending physician important information: Which treatment was successful in this specific case? Which were less effective? It is also important to involve patients in this process. “The recommendations of a CDS system should not only be intelligible for doctors. They should also be communicated to the patient,” says Andrea Schenk.
This applies in particular to the use of AI algorithms, where it is essential that conclusions don’t appear to come out of a black box. They should be transparent and comprehensible for the user. “The human being must remain at the console, so to speak,” stresses Markus Wenzel. “We view CDS systems as tools for managing ever-increasing complexity to even be able to support all involved parties during multidimensional decision making. Using such systems gives us hope that physicians will have more time for their patients in the future.